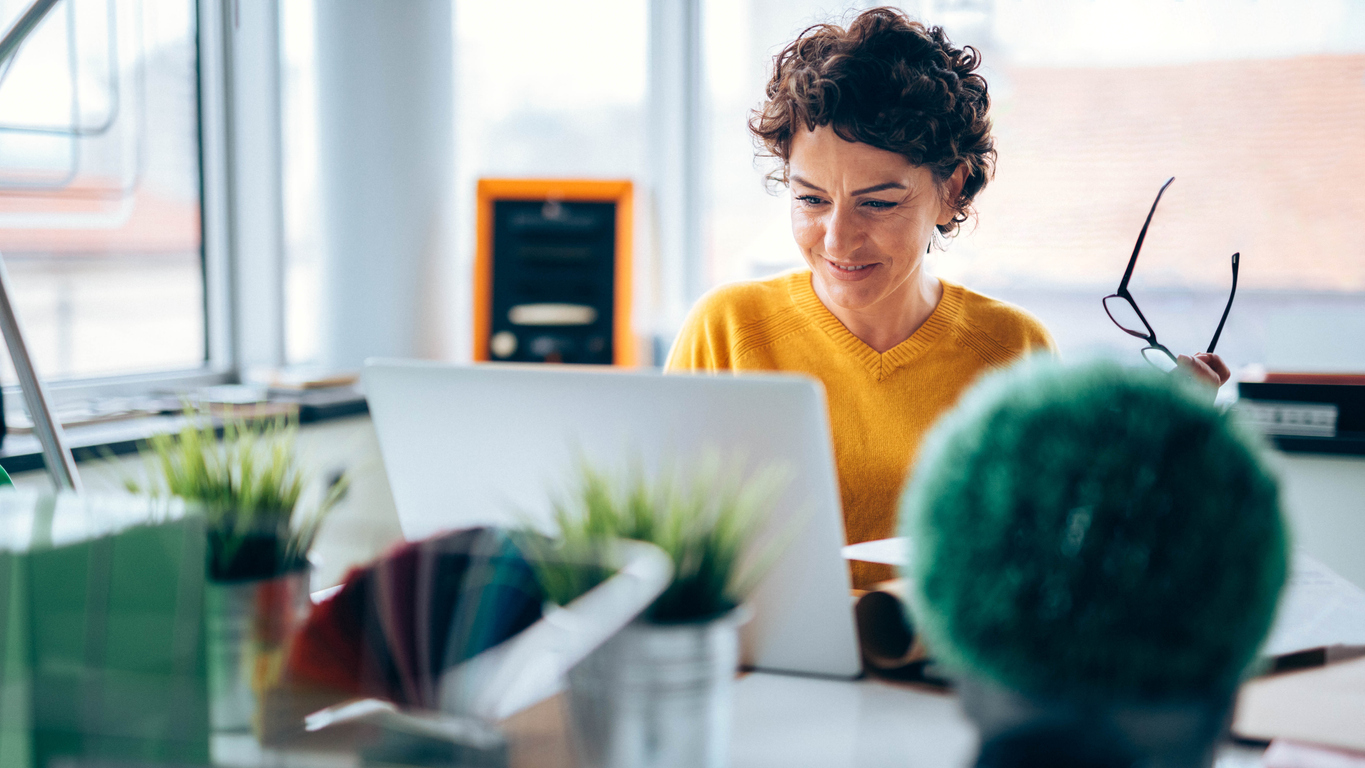
How Clean, Informative, Explainable Interfaces Increase Trust with AI Products
As a SaaS company, you might think that you most frequently communicate with your customers through your sales, support or customer success teams. However, in reality, most communication happens through your product’s interface. Your users interact with the product every day, and that is where they are forming their impressions of whether they trust your company. If they’re frustrated with the experience because of bad design, a lack of transparency, or poor communication, it’s hard for them to build trust with your company. Conversely, if you are able to show that you have anticipated your users’ needs around trust, you can make your product even more sticky and decrease your risk of churn.
With products that leverage AI and machine learning, there are additional challenges around trust that your user experience team should consider. The newness of the technology, the use of personal data and explainability – understanding why these products reach the decisions they do – all play into how users think about trust with AI products.
By focusing on trust, you can create a best-in-class user experience, that not only enhances the value of the product, but also addresses any questions of comfort. To do so, you have to build the product around your users. While your product is leveraging AI, your users are only human. Adopt a user-first mindset, maintaining a consistent voice and persona, and providing contextual explanations with the appropriate level of detail.
Remember that most users will want to use your product to complete their tasks as quickly as possible. By providing clean, informative, explainable interfaces, you’ll make it easy for them to find the information they need to get their jobs done.
Adopt a user-first mindset
The best interfaces are intuitive, clean, context-aware, and personalized, while making it easy to drill deeper if required. That means they understand who the user is and what they’re trying to accomplish. The best products use what they know about their users to clearly present the information that is most useful in those circumstances.
6 Ways to Get User Insights to Build Trust into Your Product’s Interface:
- Understand the user’s experience.
- Create two-way communication between designers (and writers) and users.
- Gather user input during the design phase.
- Ensure user testing is part of the process.
- Avoid relying on narrow strategies for collecting data.
- Don’t just rely on quantitative data, seek opportunities to hear first hand what customers want.
When you’re immersed in the day-to-day of building software products, it’s easy to forget about user experience. But you can gain valuable new perspectives by taking time to understand how people see your product, especially when they are new or infrequent users. Think about, for example, whether you’re using jargon. Terms that you use every day might miss the mark with your customers. Your users might have specific questions about where to find information about how you use the data they provide.
You can gain these insights through a few changes to the design process. First, set up opportunities for designers and writers to talk to users as frequently as possible. Gather user input during the design phase and ensure user testing is part of the process. Through an open two-way dialogue, your designers can acquire a deeper understanding of how people interact with your product and see where their frustrations lie.
Try to gather as many data points as possible and include mixed methods for collecting data. Relying solely on customer satisfaction polls or net promoter scores is limiting and won’t supply the narrative you need to make informed decisions. Be sure to include qualitative questions in your surveys. Go onsite to see customers using the product live, listen in on call center operations and hear first hand what customers request, run live focus groups and, of course, go on sales calls to see the process, hear the discussions and needs that prospective customers have to help you design better solutions.
All of these will provide different perspectives and data points on product usage and, through feedback loops, you can incorporate your learnings back into the product.
Maintain a consistent voice and persona
Once you are collecting data about your users with the techniques above, use it to personalize the experience of your product. Any information you present in your interface is part of a conversation. If your brand’s persona is friendly and informal, maintain that tone in your interface, including explanations and support literature. If you have different levels of users, you might need to adjust the pitch of your language to reflect that. For example, a technical user would understand terms a non-technical user might not.
What you communicate to your users via your interface is part of an ongoing relationship. Think about how you can use the data you gather to personalize your interactions. If, for instance, your users have different levels of expertise, adjust the tone of your language to reflect that. If you have data to show what they are most likely looking to accomplish from previous interactions, display these workflows prominently.
Provide contextual explanations with the appropriate level of detail
Until your solution can automate processes completely, you will likely need to provide some form of explanation alongside your results to help your users understand and contextualize the information. Most people will not take the results of a system that’s able to make complex decisions at face value without some ability to figure out what’s going on and why a particular decision has been reached.
Tim Miller, an associate professor at the University of Melbourne, and a researcher in the area of human-friendly explanations said: “There is so much research going into explainable AI methods and tools right now. However, very little of this acknowledges that it is ultimately everyday people who will use these tools.”
Building explainability into the product will come at the design phase. Teams will need to consider the type of decision that will be made, the people who will use the product and the types of questions those people will likely want to ask.
The best products will then be designed to make it clear what questions can be asked about the decisions and how the explainability functionality works and what its limitations are. To do this well, teams may need to incorporate social scientists, interaction designers and communication designers. Where required, consider adding a conversational interface to your explainability functionality to allow for a broader range of interactions.
“If we continue along the current path of just having AI experts researching, developing and evaluating explainable AI, there is a serious risk that our explainable tools will satisfy only those experts, leaving end users none the wiser,” said Tim Miller. “This is a topic that requires a truly interdisciplinary approach between computer scientists, social scientists, and interaction designers.”
When giving explanations, it is good practice to provide the right amount of supporting information for the situation, acknowledging that this might differ by user.
Let’s take, an expert who is interpreting the results of a model to provide their own opinion, like a doctor or a lawyer, as an example. They will benefit from richer context about the underlying model mechanism used to reach a decision to help support their own decision-making. This might include the ability to browse summarized input data to help the user understand the evidence base. If they are expert enough to have reached their own opinion, they will likely appreciate the ability to ask why the system did not reach the same decision.
Human-friendly explanations
Nothing is more frustrating than receiving an explanation that doesn’t help you understand. Well, perhaps no explanation at all. Here are some commonly accepted best-practice recommendations for providing explanations:
- People prefer short explanations, give a maximum of three reasons – don’t overwhelm them with too much information. It is harder to know what action to take with an exhaustive list of explanations. This may involve summarizing a list of reasons or selecting the two that had the most bearing on the outcome.
- People don’t want to know why they receive a particular prediction, but why they received it instead of another prediction. Use contrasting explanations: “You would have been accepted if you had done this instead of that.”
- If one data point is abnormal and has an outsize impact on the outcome, it is useful to provide this as a reason.
- Though you will have to omit details by being selective, humans need to be sure that the outcome is truthful. If they feel that the result is unfair, they will lose trust.
- An explanation is part of a conversation. If your interface is friendly and informal, maintain that tone in your explanations. If you have different levels of users, you might need to adjust the pitch of your language to reflect that. For example, a technical user would appreciate more detail than a non-technical user.
- Give preference to explanations that do not challenge existing beliefs. Due to confirmation bias, your users will ignore this information.
- Keep the conversation general and probable. Don’t go into the specifics.
Getting started
An interactive, fully explainable interface is the end goal, but you can get started down this path by reviewing new interface designs with a range of users at different technical levels. Observe them using the product: Do they all intuitively know where to go? What are the common questions? Also, do you provide that information readily? Bring different members of your team into these reviews so that you get a diverse set of perspectives and expertise on how you can use this information to start thinking about your explainability roadmap.
Read more like this
Redefining legal impact with the team at Darrow
When we think about legal tech software, we think about value add…
Testing LLMs for trust and safety
We all get a few chuckles when autocorrect gets something wrong, but…
Introducing Georgian’s “Crawl, Walk, Run” Framework for Adopting Generative AI
Since its founding in 2008, Georgian has conducted diligence on hundreds of…