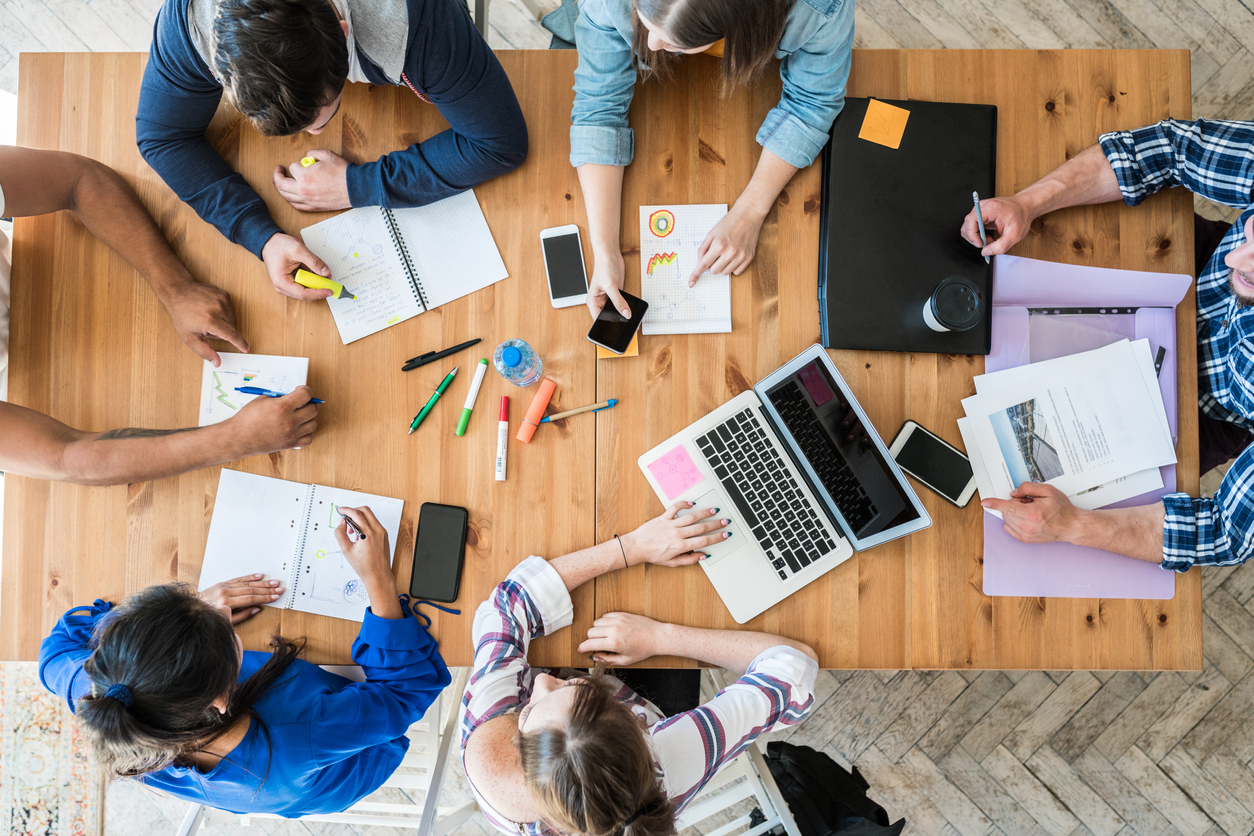
Six Key Insights on Product Management From Virtual Scaletech 1.0
San Francisco-based Beckie Wood joined us for our first Virtual Scaletech AI Product event on May 20. Beckie spent 12 years at music streaming pioneer Pandora, before that she started her career in investment banking and equity research. She joined Pandora when it was a scrappy 60 person company and got to work on early personalization and recommender capabilities all the way through until 2019 when she led a 65 person cross-functional team. The following six insights are based on the excerpts from Beckie’s presentation and Q&A with Alistair Croll during the event.
1. Be Clear About What You Are Building
When you think about product strategy and what you want to build, the most important thing is to have an important problem that you’re solving for a ton of users and that you solve it in a unique and differentiated way. It’s critical to be extremely clear and concise when considering your product strategy. My preference for mapping that out is to use V2MOM: Vision, Values, Methods, Obstacles, and Measurement.
Many good companies already know what they’re building and why they’re building it, and the best companies also have a clear mission. However, many startups find it tough to find great product-market fit from the beginning.
I sometimes think this problem arises because technology offers so many possibilities. People take more of a scattershot approach to using AI and ML to solve the problem. The key is to come back, refine, and reiterate your mission and vision so that you and your team can align on what you’re building and why.
2. “Humans For Quality and Machines For Scale”
At Pandora, we used to have the saying “humans for quality and machines for scale.” Our curation team and musicological experts were strategically placed to think about ways to make the dataset better. That was important because—as with all large datasets—we knew there would be issues with the data; that there would be data that needs to be scrubbed and people would need to make decisions that overrule the machine learning systems.
A great example I have for this is genre stations. Pandora has different types of genre stations. One being classic rock, another being jazz, and another being the 1980s station. From a strict machine learning perspective, the 1980s station might only play music literally released in the 1980s, or maybe it should only play music that was popular in the 1980s. In this case, humans need to step in and make sure the system is including the music that the system might miss by itself.
3. Traditional vs. AI Product Management and Hiring
Traditional product management is pretty clear. Generally, you’re figuring out what you’re building and why you’re building it, looking at front-end use cases all the way to back-end use cases, partnering with engineers and getting things built.
With AI products, the focus is on creating various types of recommender or prediction systems and partnering very closely with the machine learning scientists to develop and train these systems.
My recommendation is to focus on hiring product managers that are back-end focused for AI product management roles. These people will be more technical and understand modeling and basic machine learning technologies.
If you’re hiring early on in a company’s life and you’re just hiring a single product manager for AI, it’s really valuable to have somebody with experience working on recommenders. This in practice can be difficult and so if it’s not possible, work to find someone with a data background, who’s been a data scientist, or understands the complexities of what a truly complex system might look like.
Lastly, when interviewing candidates keep an eye out for strong analytical capabilities, an aptitude or interest in coding, and the ability to navigate different types of personalities and needs.
4. “WTFs” Are Metrics Too
Content companies like Pandora and Netflix have the luxury of suggesting a bad show or a bad song and it not being the end of the world. In fact, if the customer gives a thumbs down or direct feedback you have a definite reaction on which direction not to go in the future. However, not every company has this luxury. For example, this situation is very different if you are building self-driving cars.
For any company using AI, it’s essential to think about those trade-offs; how problematic it is if things fail. At Pandora, we used to call these “WTFs”. Treat these “WTFs” like any other metric. Track how often those situations occur and if it’s appropriate for your product to have these learning moments.
5. Christmas Is Cancelled
Back in the early days of Pandora, before the days of genre stations, certain stations acted as a genre station. For example, there was a station called Christmas, which we hadn’t created, that was populated with songs from a rock band called Christmas. The reality was that people who typed in Christmas expected Christmas songs to be playing. Over time, people thumbed down all of the songs by that band and continued to thumbs down all songs that played until Christmas songs started playing.
Pandora hadn’t built Christmas stations and this was a result of the AI system. This was an eye-opening experience for us as it was a clear use case that we should have known about and solved for. It’s strange to think that although you designed the system, you have no idea what is actually going to happen.
6. Balancing Quality of Results With the Magic of Experience
At Pandora, simplicity was one of our core principles. This made it tough to make any explicit changes unless we could prove it was significant.
This is an ongoing challenge for people who design products. You want to keep things as simple as possible for the user, but when you allow people to give more explicit signals you’re making the system significantly more capable.
A balanced approach is to send a survey to someone if you think they’ve had a bad experience. This is a targeted approach that doesn’t break the simplicity or the magic of the experience but does try and elicit feedback for improvements.
If you enjoyed this read and you’d like to be invited to our next Scaletech AI Product event in July, shoot katie@georgian.io an email.
Read more like this
Cloud Spend Management: A Guide for Startups
Over the past several months, CoLab executives and customers have told us…
How to Use OKRs to Unlock Your Company’s Potential
You’re probably familiar with OKRs — Objectives and Key Results. OKRs are…
Team Profile: Azin Asgarian, Applied Research Scientist
What do you work on at Georgian? As part of the R&D…