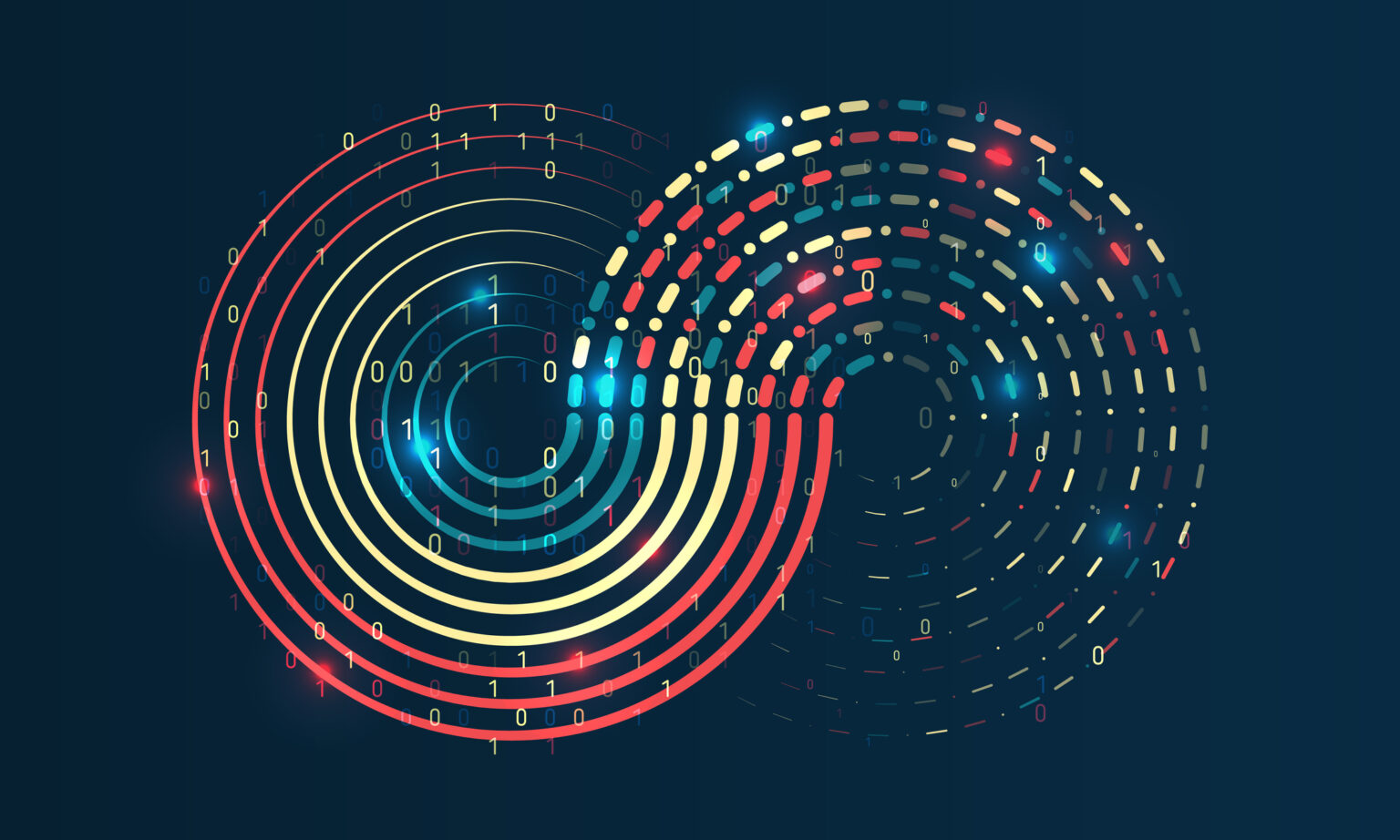
Generative AI’s Overnight Success Was Years in the Making
Since Georgian launched its Applied AI thesis in 2017, we’ve seen an explosion in AI applications for software companies. With the rise of better models and better tooling, AI has become ubiquitous in many businesses. However, even with mainstream use of AI, very few in the industry expected the explosion of public interest in generative AI caused by ChatGPT.
Since launching in November 2022, ChatGPT has created a firestorm of public and media interest in — and debate about — the future of AI, bringing the term ‘generative AI’ firmly into mainstream use. While we believe generative AI could revolutionize content creation by enabling rich, personalized content to be created in seconds, we believe it is important not to focus only on the ‘generative’ part of generative AI. There is much more to this technology than generating marketing content or writing essays.
For example, Georgian AI, our applied AI research lab, has already supported Georgian customers (portfolio companies) to deploy real-world applications of large language models that showcase generative AI’s use cases beyond simulating human writing. What all these applications have in common is the use of transformer-based language models — a model standard in generative AI applications. These models hold enormous potential, encapsulating an understanding of human language and knowledge in a way that wasn’t previously possible.
We believe generative AI has the potential for a wide range of use cases, including intelligent automation of business workflows, accelerating human learning and science, knowledge management and human-computer interaction (as shown by ChatGPT).
Generative AI allows people to create personalized content in seconds, ushering in a new paradigm for business. However, in our view, the technologies on which it is built — large language models (for text) and diffusion models (for images) — enable broader use cases beyond the ‘generative’ ones we see now.
This blog gives an overview of generative AI, how it works and important developments in the field. If you’re looking for insight of real-world applications, in the coming weeks, we’ll share a blog on opportunities and challenges in generative AI.
Key terms in Generative AI
Throughout this blog, we’ll be discussing the models and techniques underlying generative AI. In case you get a bit lost, refer back to this list of key terms we put together for a reminder.
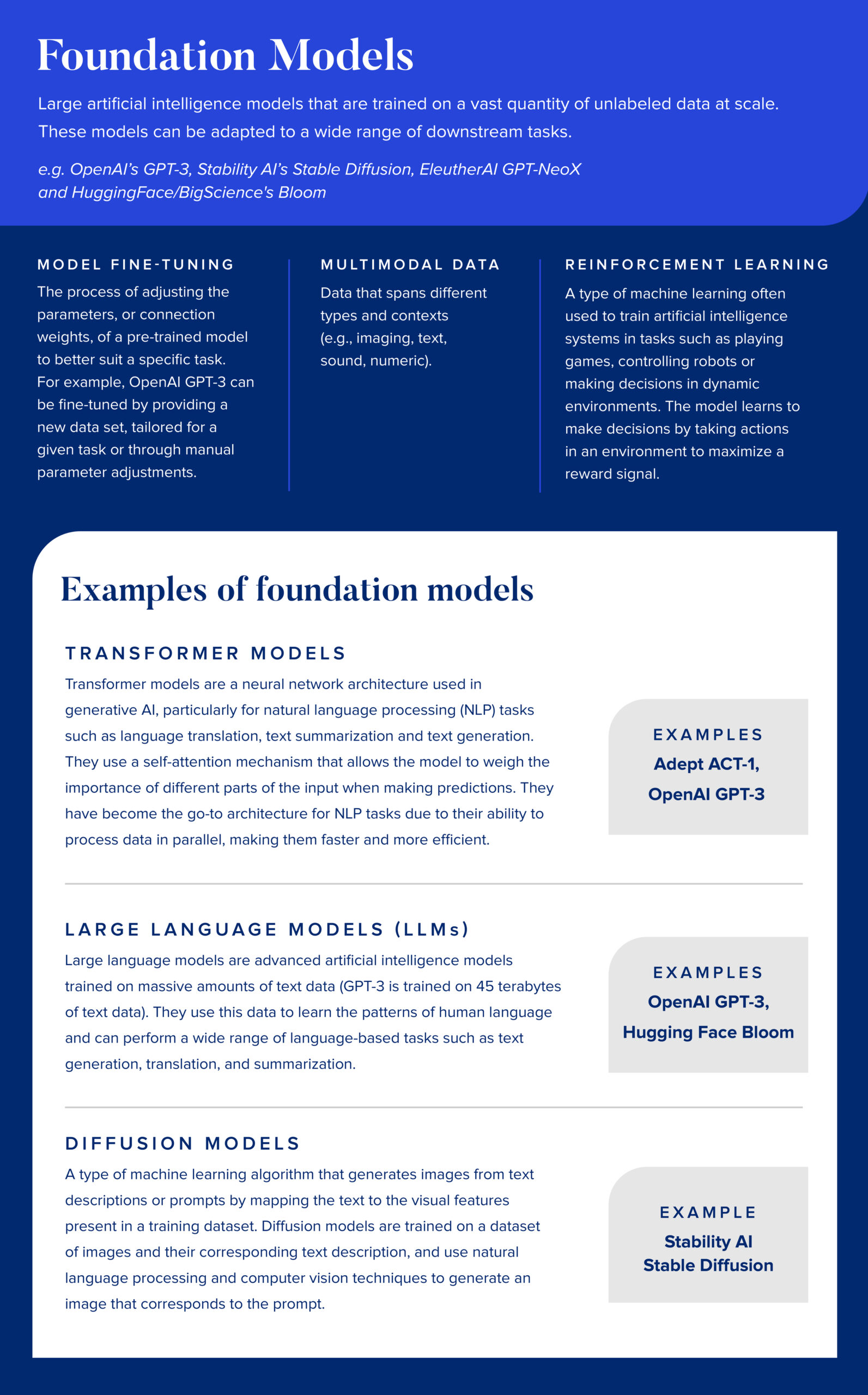
How Generative AI works
The recent success of GPT-3 and ChatGPT comes from the use of ‘transformers,’ a deep learning approach that’s undergone significant development over the last five years. As Nvidia puts it, transformers are neural networks that learn context and meaning by tracking relationships in data, like words in a sentence.
Transformers were first introduced in 2017 by the Google Brain team, and it is now the go-to approach for solving natural language processing (NLP) — the ability for computers to understand human language — and machine vision challenges such as object detection for autonomous vehicles. Transformers enable models such as GPT-3 and GPT-Neo, and the image diffusion models DALL-E and Stable Diffusion.
We believe generative AI could be a game-changer for many businesses. In the medical industry, for example, generative AI has been used to simulate drug discovery and create synthetic medical data, and could be used to generate 3D models of organs for surgical planning. Creative industries have used generative AI to create art, music and literature. Meanwhile, in finance, generative AI can help with complex fraud detection and knowledge base search so important information is not missed.
With its ability to produce new and diverse outputs, we believe generative AI can be an invaluable tool for solving complex problems across various fields.
How Generative AI has evolved
ChatGPT’s development comes from years of research into generative AI models and the chatbot’s use of an ML approach called reinforcement learning (RL). In an RL system, an intelligent agent (in AI, this is an autonomous program) interacts with and learns from, its environment. In the case of ChatGPT that ‘environment’ is you, the user.
Improvements over recent years in generative AI model performance are, in our opinion, due to several factors, including:
More data: With the explosion of digital data in recent years, there is more data available to train generative models. This data availability has enabled models to learn from a larger and more diverse set of data, which, in our view, can lead to better performance.
More advanced algorithms: There have been numerous advancements in generative modeling algorithms in recent years, such as Generative Adversarial Networks (GANs), Variational Autoencoders (VAEs) and transformers. These models are better able to capture complex relationships in data and generate high-quality samples.
Better hardware: The availability of more powerful hardware, such as GPUs and TPUs (Tensor Processing Units), enables researchers to train larger and more complex models. This ability to train larger models has led to significant improvements in the quality of generative models.
Transfer learning: Generative models can now benefit from transfer learning, where models pre-trained on large datasets can be fine-tuned on smaller, task-specific datasets or on a set of prompts designed for that task. By leveraging the knowledge learned during pre-training, the generative AI can often produce higher-quality outputs with fewer data and training time.
However, for many years, despite advances in the performance of multiple large language models (LLMs), including GPT-3 (on which ChatGPT is based), BERT and GPT-Neo, these models did not catch the attention of the general public and media. In fact, the general public only started paying attention once OpenAI made the capabilities of GPT-3 available via an easy-to-use interface that anyone with an internet browser can make use of: ChatGPT.
In our view, the ease of access to AI capabilities opened the broader public’s eyes to generative AI’s possibilities.
Where do we go from here?
Currently, a lot of public discussion on generative AI focuses on generative text content, such as high school essays or marketing content (which use LLMs), or on the generation of digital art using Latent Diffusion Models (LDMs) like Stable Diffusion and DALL-E.
However, in our view, generative AI has applications that go beyond content generation.
For example, generative AI could accelerate AI adoption and conversational interfaces, and companies can leverage these foundational models and combine it with their unique data assets. In fact, in our opinion, the wide availability of generative AI models from a variety of vendors and open source projects, and the ability to fine tune those models with proprietary data, means that software companies who have strong data moats stand to gain the most. Increasingly, we expect to see LLMs trained on proprietary data and information.
For startups especially, it may not make sense to train LLMs all the time because of high costs and impacts on the environment. However, there are ways to use pre-trained LLMs in a pragmatic way that can help companies start their generative AI journey. Some use cases like summarizing information or expanding on information based on a few bullet points, can happen out-of-the-box with these LLMs. Another example would be using other NLP techniques, like search, that can augment your prompt with your data to facilitate tailored conversations for your customers.
Beyond conversational applications, generative AI also has the potential to automate designs in engineering, creating variations at a faster pace, optimizing materials and predicting how a design will perform under different conditions, accounting for factors like demographics, preferences and physical characteristics. In addition, testing iterations may become more efficient where generative AI can digitally simulate the performance of a product under different conditions, providing insights that would be difficult or impossible to obtain through physical testing. Finally, design processes may become more accessible to non-experts as complex process points are automated, and people can interact with the AI thanks to user-friendly interfaces that use natural language.
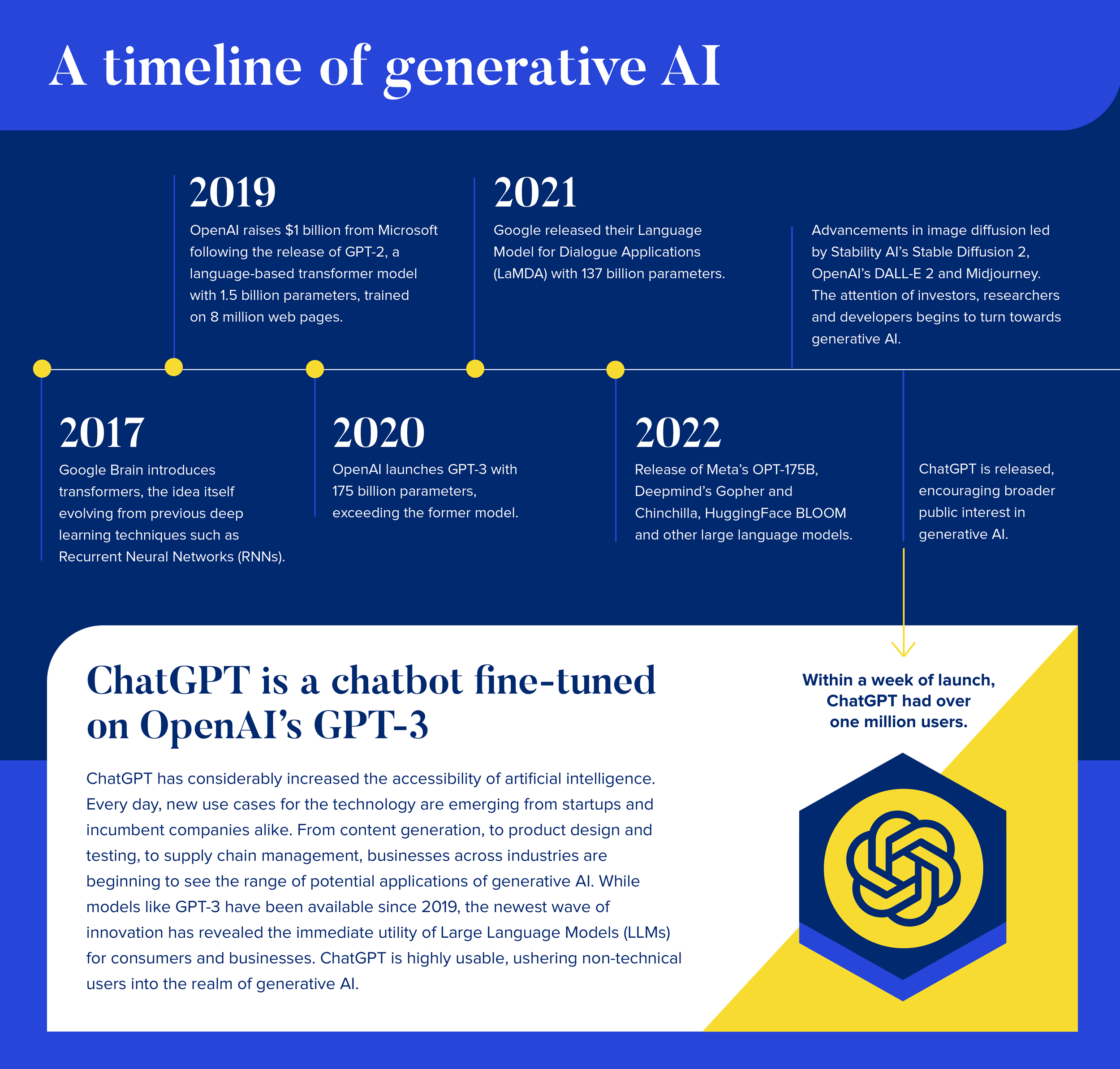
Why we’re excited about SaaS + Generative AI
In our opinion, some of the most exciting generative AI applications will come from existing SaaS markets where firms apply the technology to their unique data moats. In other words, we believe that generative AI has the potential to be an innovation accelerant for SaaS companies.
ChatGPT’s accessibility to the public may keep a lot of public focus on text-based applications in the short term. In our opinion, there is significant potential to tackle a wide range of challenges including workforce automation, knowledge management, drug discovery and engineering design — problems that have been very difficult to solve to date with existing technologies.
As LLMs become more accessible and integrated into businesses, we think it will change the tech industry’s idea of what makes a differentiated company in the same way that using machine learning is considered table stakes today. One critical question that remains is how businesses integrating generative AI can maintain a competitive moat as access to AI technology becomes more democratized.
In our next blog post, we will dive into the specific business challenges and opportunities in adopting generative AI and staying competitive.
Looking for content on what’s next in generative AI and other emerging technologies? Subscribe to the Georgian newsletter for curated resources and Georgian insights on the latest tech trends.
Read more like this
Agentic AI and the Rumors of SaaS’s Demise
Introduction: A Conversation About Big Shifts When I joined Tobias Macey on…
Why Georgian Invested in Island (Again)
Island, the developer of the Enterprise Browser, emerged from stealth in early…
Why Georgian Invested in Render
We are pleased to announce Georgian’s investment in Render’s $80M Series C…