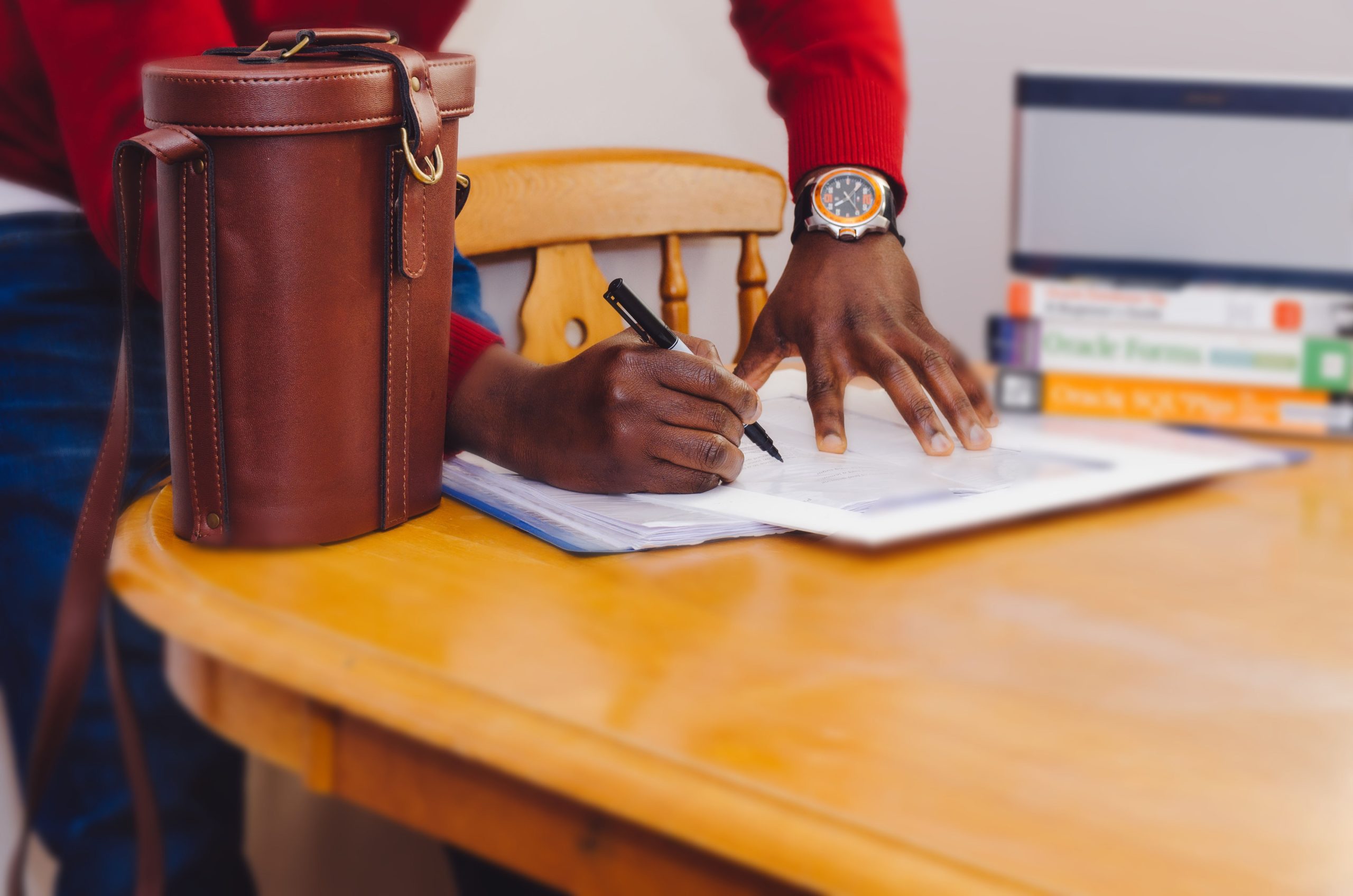
Building ML Teams Resources
We’ve pulled together twelve top resources to help you set the vision, prioritize opportunities, and hire a remarkable team. To get started with AI, there are critical steps that must be taken to ensure success.
Understanding the feasibility of the opportunity comes first, and having the right team is next. If you are considering AI for your business, these resources are an excellent place to start.
Strategy Resources
1. Georgian’s Principles of Applied AI
This white paper introduces our ten principles of applied AI and lays out a detailed framework for getting started. The paper shows how you can avoid common mistakes and provides you with a method of measuring your progress. You’ll gain a better understanding of what business AI problems can solve and what it would take for you to implement a solution.
2. Andrew Ng’s AI Transformation Playbook
Andrew Ng’s AI Transformation Playbook delivers insights gleaned from his days leading the Google Brain team as well as the Baidu AI Group. By following the Playbook, Ng says that any AI-focused buisiness can gain the same kind of momentum and success he details here. The Playbook has a strong focus on building an in-house AI team as well as how companies can leverage it to their best advantage.
3. AI Canvas Presentation
The AI Canvas is a methodology that helps you build business cases for AI projects. It will prove your concept and ensure that all angles have been considered before moving forward. The bottom line is, the feasibility of your AI project should always be front and center. Its real superpower is helping you sell your idea to others – whether they are executives, other team members, or the end-user. Loaded with plenty of scenarios and examples, the AI Canvas is a template you can use and modify according to your specific needs and use cases.
4. Coursera
If you or your team needs to gain a better understanding of AI, neural networks, data science, machine learning and deep learning, this course is a great place to start. Taught by Andrew Ng, the course focuses on the business aspects of AI, how to work effectively with AI teams, and how best to develop an AI strategy within your company.
5. How CEOs Can Tap AI’s Full Potential
An effective AI strategy requires people and technology to work together. This framework outlines the steps that must be taken to identify the opportunities, visualize the result and ensure buy-in from your teams.
Hiring Resources
1. How To Consistently Hire Remarkable Data Scientists
Jeremy Stanley is the Chief Data Scientist at Sailthru and he knows a thing or two—not only about data science but also about hiring the minds behind it. In this article, you’ll find practical insights on recruiting, potential hang-ups you might encounter and how to set objectives to assure a successful process. Read this article if you want to reduce your cost to hire and improve your data scientist hiring success rate.
2. How Does Airbnb Hire Data Scientists?
Here is some fantastic insight on how one of the world’s top AI platforms hires data scientists, how their approach has evolved over the years, and what they’ve learned from their mistakes. It is a fascinating glimpse into one company’s approach to data science success.
3. Bighead: Airbnb’s Machine Learning Platform with Atul Kale
If you want to dive even deeper into what makes Airbnb tick, Bighead should be your next stop. Bighead is the machine learning platform that powers Airbnb’s AI. As for Atul Kale, his work focuses on understanding how large companies are using AI, machine learning and deep learning. This podcast interview with Kale covers a wide range of topics, including the ever-present question of how best a company can scale to support more data-driven objectives.
4. Essential Skills for Every Data Specialist
Jobs in data-related fields are growing in demand, day by day. In AI and ML, like all technology, platforms, languages and approaches come and go. This article provides an overview of the essential skills and languages today’s data specialists need to stay relevant.
5. How to Become More Marketable as a Data Scientist
Staying on top of current industry trends is the best way to be marketable in any niche. This post outlines current trends in data science and the skills that stand out to potential employers. Based on actual job postings, the stats in the article represent what top employers are looking for.
6. Are Successful Data Scientists Hired or Trained?
In 2018, Gartner released a study that suggested 85 percent of data science projects will fail. That means the other 15 percent were, hopefully, wildly successful. What did those (successful) projects have in common? Apparently, it has a great deal to do with whether your data scientists were trained or hired. While it would seem to make sense to hire someone who already had the expertise, for some situations, it might be better to train from within. After all, no outsider knows your data better than you do.
7. How Scouting an AI Engineer Should Change Your Hiring Strategy
Hiring an AI engineer is not like any other hiring process. This article encourages you to look beyond the obvious, focus on results rather than experience and learn how to compete with the likes of AI mega-giants like Facebook and Google. Ultimately, it’s about transformation. And with the demand for AI talent increasing at an exponential rate, you need to figure out not only how to scout for new blood, but how to offer what the competition can’t to a prospective new hire. Which is more acceptable?
Read our guide to building effective ML teams
This post is an extract from our guide to building ML teams.
The full guide contains:
- A framework to go from setting a vision through to launching ML products with the right people, processes and tech
- Tips for hiring top ML talent
- Job descriptions and interview questions for common ML roles
Read more like this
Why Georgian Invested in Glooko (Again)
We are pleased to announce that Georgian has led Glooko’s $100 million...
Why Georgian Invested in Coder
We are excited to announce that Georgian has led Coder’s $35M fundraise…
Why Georgian is Investing in SurrealDB
The proliferation of unstructured data has, in our view, made building modern…