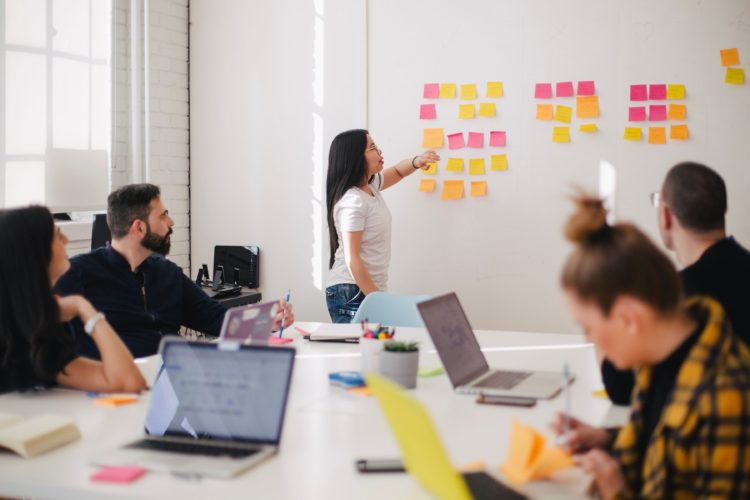
Which ML Positions Should You Hire First? Five Machine Learning Experts Discuss.
When building a new machine learning (ML) team in your startup, one of your most important decisions is which positions to fill first, with limited resources. Hiring the right team can help build your product and scale at your desired pace. There is always room to grow the team as needed from this solid base.
At our Scaletech AI Product Series event in July, we asked five ML experts which hire they would make to build out their own machine learning team. Most panelists agreed a data scientist is the obvious first hire, but there are other positions to consider.
Your company will need a data scientist to research, develop, and test your machine learning (ML) models. A data engineer will help build and improve the data pipeline since data fuels the ML model. A product manager can help decide which features engineers should build for the end-user, with a strategy to take the product from concept to reality.
As you build your team, who should you hire first?
Data Science Is Key
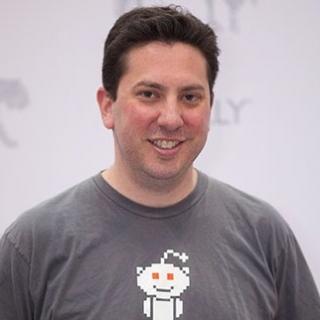
Jeremy Edberg is the CEO and Founder of MinOps, an infrastructure scanning and improvement tool. He was previously an engineer at Netflix and Reddit
“I would definitely want a data scientist on hand, ideally one who has some background in operating data science platforms: somebody who knows how to manage large data sets, move them around, that kind of thing. If you’re talking about early hires to a startup that’s going down the AI path, you’re going to want somebody who knows algorithms, knows data science, and can manage moving data around.”
“The seventh employee at Airbnb was head of data science,” Dr. Theresa Johnson pointed out.
Your Team Needs a Data Engineer
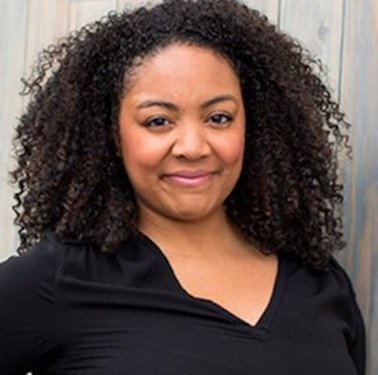
Dr. Theresa Johnson is the product manager of payments and former data scientist at Airbnb.
“Assuming we were starting from scratch with no data scientists, no ML engineers, nobody with this background in the startup, maybe just general software engineers, I’d firstly hire a super rockstar data engineer, because I fundamentally believe you need clean data. Working data pipelines are the whole bread and butter behind getting to an artificial intelligence (AI) or ML model. Do you have the right data? Is it clean? Is it continuous? So, I would want data engineering with some data science skillsets layered on: sort of full stack, but centered on data engineering.”
“Building a data pipeline is difficult,” Jeremy added. “There’s definitely companies out there trying to make this easier, but there’s no software to take it all the way from here to here. You’re going to have to customize it. You’re going to have to inject things in the middle of the pipeline. It’s a huge undertaking.”
“At Netflix,” Jeremy continued, “data science was a huge chunk of the engineering work. We had almost as many people running the platform as we had to do the data science. I’m sure it’s the same everywhere else too. It’s a huge involvement to build the pipelines.”
Product Managers Are Needed to Quarterback
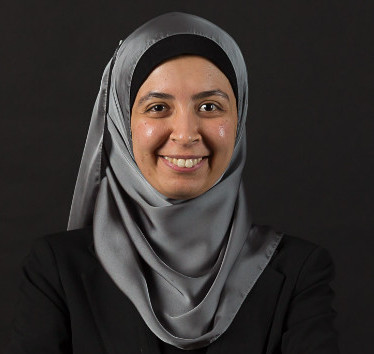
Manager at Loopio.
Nahla Salem is a Senior Product Manager at Loopio, using ML to streamline the request for proposal (RFP) process.
“Startups who want to adopt AI should hire a product manager. I guess my answer is biased.” At Georgian, we tend to agree. A product manager can help to validate market problems, create product requirements and manage the roadmap. You may be able to find a senior scientist who can take on the PM role temporarily.
Nahla continued, “My next hire would be a data scientist, someone who has a good understanding of how to bring models into the user’s hands, in terms of experimentation and taking feedback.”
UX Is Important
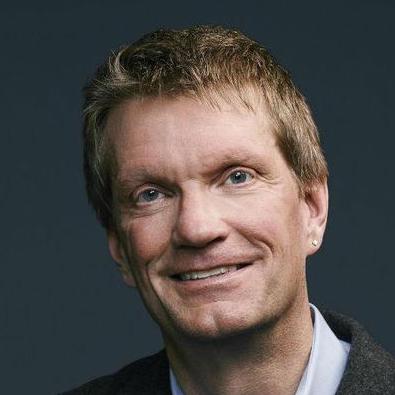
Mike Olson is a board member and former Chief Strategy Officer at Cloudera, an enterprise data cloud.
“I’m going to steal Jeremy’s answer,” he said. “Then, I’m going to tell you my second hire is a UX person, someone to figure out how to put the answer in front of a decision-maker in a useful format. UX is an overlooked part of the computer industry.”
You May Need a Liberal Arts Major
Theresa described working in Airbnb’s anti-discrimination team. “Getting accepted was harder as a black guest than it was as a white-looking guest. It’s important to re-examine how our own algorithms are potentially exacerbating this. Are we popping things up into the search results that disfavor certain hosts and guests? Are we showing certain reviews that favor or disfavor hosting guests? How can we correct that?”
“The Anti-Discrimination team was built to address this issue,” she explained, “to actually lower the acceptance rate gap, and tie that back to the business fundamentals. If you’re not accepting guests, you’re making the world less inclusive. Your belonging is suffering, and Airbnb’s business is based on belonging, so Airbnb’s business is suffering. Once we tied all that together, the problem is starting to get better”.
Mike remarked, “It’s important for us as technologists to consider how technologies are applied and whether the outcome is ethically acceptable, in addition to being profitable. A lot of the problems we’re seeing in the world right now are because big platforms chased engagement without regard to outcome.”
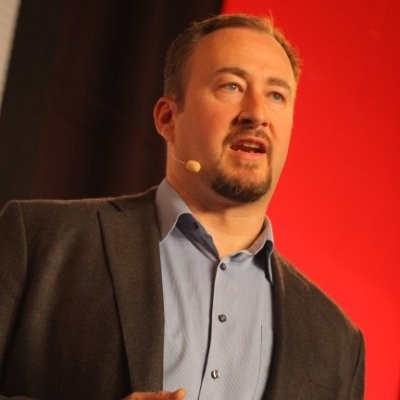
Moderator Alistair Croll, the founder of Scaletech Conference, wondered, “Maybe the third or fourth hire should be a liberal arts major with a background in thinking things through. I think there’s not enough of that. There’s going to be a dramatic shift in who we hire for.”
Your Key Takeaways
Our panel experts agreed your first or second hire should be a strong data scientist or data engineer. Other positions like product managers and user experience specialists help keep the end goal in focus, creating a solution your users will want to use. It’s also important to ensure that early hires keep ethics in mind, helping avoid building bias into the technology.
As your team grows, you may also need an ML architect, a quality assurance (QA) engineer, data analysts and annotators and a designer focused on user experience. An ethicist can help you avoid unintended consequences of your AI product.
If you’re building your first AI product and are thinking through how to set the team up for success, take a look at Georgian’s Guide to Building ML Teams.
Read more like this
Agentic AI and the Rumors of SaaS’s Demise
Introduction: A Conversation About Big Shifts When I joined Tobias Macey on…
Why Georgian Invested in Island (Again)
Island, the developer of the Enterprise Browser, emerged from stealth in early…
Why Georgian Invested in Render
We are pleased to announce Georgian’s investment in Render’s $80M Series C…