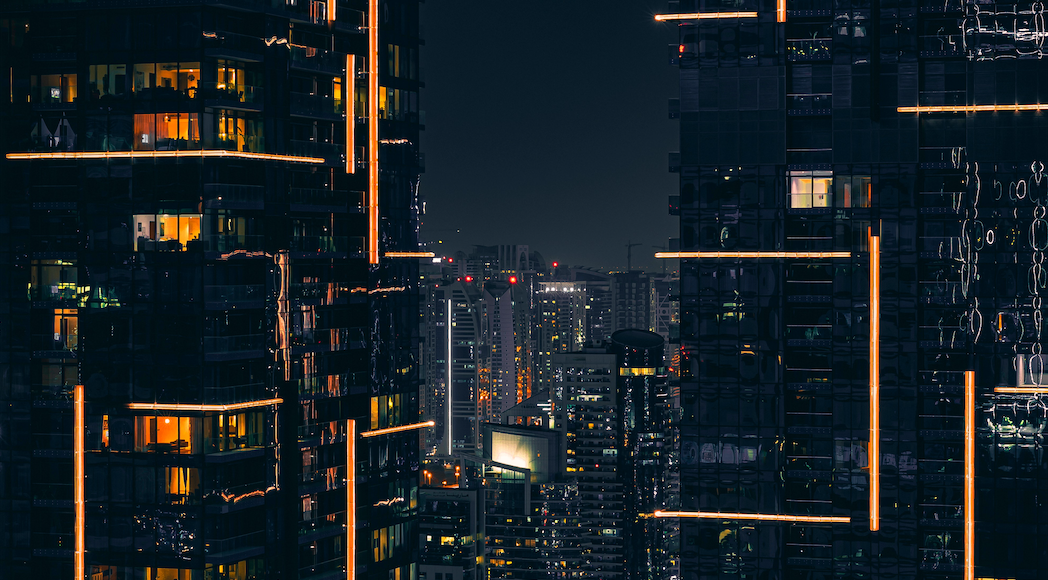
A Guide To Scaling Your AI Product
Artificial intelligence (AI) is revolutionizing business — but that doesn’t make it valuable for all organizations looking to generate value and drive growth. The challenge is knowing when and how to use AI wisely.
Dr. Jerrold Jackson is VP and Head of Machine Learning and Data at Exos, a human performance and wellness company, where he is using AI to deliver personalization and optimize customer experience in real time. At Georgian’s ScaleTech Conference, he shared his insights on the fastest and most effective way to create value by adding AI capabilities to your products and services.
Drawing on his experience designing and implementing data strategies at Exos and in several other fast-moving industries, Jerrold provided real-life examples of when and how to scale AI in your organization, plus tangible ways to evaluate success. Below, we round up some of the top takeaways from his talk, like the right way to evaluate AI opportunities and scaling your AI product.
Is AI a good fit?
AI can help companies deliver tremendous value to their customers but it isn’t the right tool for everyone. So how do you evaluate whether AI is the right solution at the right time? Jerrold said the answer is often driven by data.
AI is data-hungry and requires constant feeding, so it is best applied in industries where data is plentiful and accumulates quickly, such as fintech or adtech, where it can be used to train and refine machine-learning algorithms.
“Many growth-stage companies don’t naturally have high data volumes or data that is dynamic enough and frequent enough to feed AI methods and systems,” Jerrold said.
That doesn’t mean an AI solution is off the table for them. There are ways that growth-stage companies can still take advantage of smaller, high-quality data sets or set themselves up to be AI-ready in the future. If you think AI will be key to creating value for your company, Jerrold recommended planning today for the data you will need later.
Finally, whether you take the plunge and adopt AI today or are planning for the future, Jerrold said companies should proceed with caution. AI systems are a major investment and results may take time. “The user experience of AI isn’t magical. The underlying capabilities take a lot of time to develop and perfect,” he advised. “Be realistic and sober-minded about that.”
How to scale AI
An organization has decided to invest in AI. How should it scale its AI efforts? Jerrold shared the following five tips, drawing from his experience bringing ML and AI capabilities to Exos, as well as in financial services and the resale ticket market.
Develop clear use cases: The first step is to identify areas where ML and AI can make a difference. If you have a laundry list of applications, or use cases, cut it down by focusing on areas where the technology will have a major impact. “One way to give ML and AI-based efforts velocity is to name and clarify the use case or use cases,” said Jerrold.
Invest time and money: Cost can be an obstacle to scaling AI. While the technologies that power AI are becoming increasingly affordable, they still come with a high price tag. Time is another consideration, since building AI capabilities and getting results takes time. Jerrold likened adopting AI to starting a new garden: “You may sometimes water it, you may invest in it and not see immediate returns and growth,” he said. “It really takes time, so be patient.”
Given the need for upfront investments in both time and month, Jerrold said demonstrating value immediately is important. He suggested shooting for small, incremental wins — a strategy he is employing at Exos.
At Exos, Jerrold is targeting areas of the business where AI can make a difference quickly, as well as areas that are likely to pay off down the road. “AI-based use cases are new but we also want to see impact immediately. I got buy-in on use cases at the outset and now have AI in the product, in the first year, driving recommendations and early personalization,” he said.
Buy vs. build: Jerrold advocated for buying AI technologies, data sets and other components over building them. Why? Off-the-shelf solutions let you quickly demonstrate how AI can create value for your business. They also allow you to test your approach before building capacity in house, refine your use cases, and overcome barriers to AI adoption, such as access to relevant data sets and trained classifiers for organizing those data.
Prioritize data quality: If you don’t have big or fast data, then prioritize data quality. Jerrold has found that data quality is one of the major factors influencing the performance and cost of an AI system, even for smaller data sets. “High data quality and focusing on the data governance aspects of that is really one of the best kept secrets,” he said.
Show incremental progress: Take an incremental approach to scaling AI and plan for that from the start. At Exos, for example, they think in terms of good, better, best. Jerrold suggested thinking about what is good enough at first to yield value, while simultaneously having a plan to get from good to better and from better to best. He also suggested aligning on metrics for success at each of those stages.
When to scale AI
Above all else, the choice of when to scale AI should be driven by speed, accuracy and scale.
Put another way, you need to be able to deliver the “magic experience the end-user enjoys,” said Jerrold. He suggested considering whether AI is the only option or whether there are other approaches that could be just as successful at doing that. “Don’t lose sight of other approaches,” he advised, especially those that could show value while you build your organization’s AI capabilities.
How to evaluate success
In keeping with the strategy of demonstrating incremental progress, the metrics you use to evaluate success should align with your short-, medium- and long-term goals.
At Exos, Jerrold aligns his data strategy with metrics like quarterly objectives and key results (OKRs) and key performance indicators (KPIs) and the company’s annual goals. He described the company’s shift from a traditional set of uses cases and slow data to new uses cases and bigger, faster data that allow them to use ML and AI to drive personalization in product.
As that shift took place, he asked himself, “What’s the fastest and most effective way I can create value today, tomorrow, next quarter and next year?”
Read more like this
Agentic AI and the Rumors of SaaS’s Demise
Introduction: A Conversation About Big Shifts When I joined Tobias Macey on…
Why Georgian Invested in Island (Again)
Island, the developer of the Enterprise Browser, emerged from stealth in early…
Why Georgian Invested in Render
We are pleased to announce Georgian’s investment in Render’s $80M Series C…