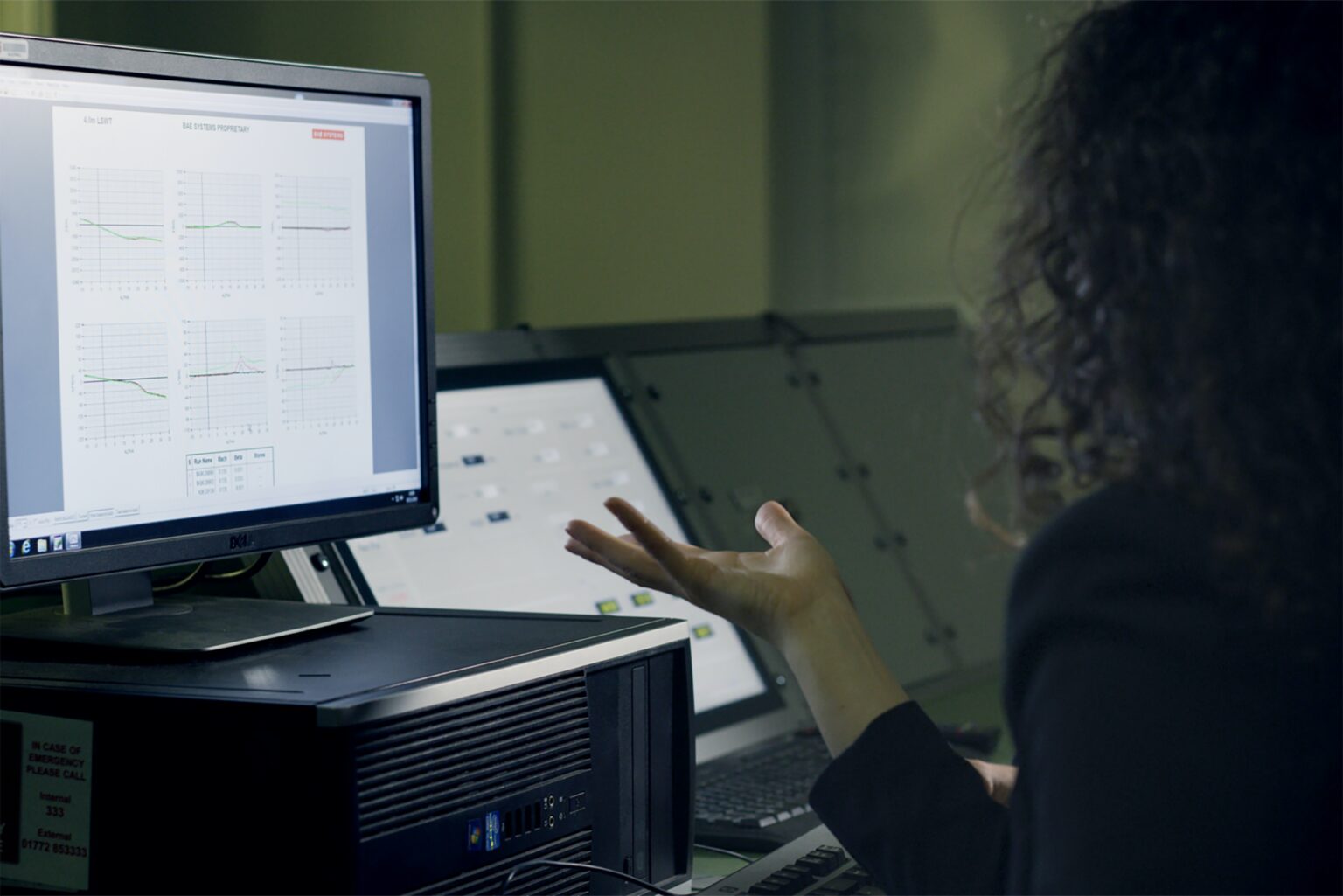
How To Find the Best AI Opportunities in Your Business
Building an AI product can seem daunting if you don’t know where to start. How do I attract the right people to build a working product or service? Do I have the right data? How do I know if using AI is the right way to solve my problems?
These are some of the questions answered at the latest CoLab Connect event on getting AI-ready. During a panel moderated by David Tingle, R&D Ops at Georgian, Georgian Head of Applied Research Parinaz Sobhani and DefenseStorm co-founder and chief product officer Edgardo Nazario explained how to overcome common challenges you’ll encounter when building an AI product.
Prioritize AI opportunities
Let’s make sure that it’s not a solution looking for a problem, but rather we identify business problems that we see.
Edgardo Nazario
You can’t effectively apply AI to your business without knowing what problem you’re trying to solve. Similar to product development in any business, you’ll have to do the work — customer calls, discovery, evaluation of pain points — to figure out the right business problem to solve.
In Georgian’s AI white paper, principles 1 and 2 explain this process of finding opportunities in more detail, which includes mapping your business processes, understanding your desired outcomes and prioritizing the opportunities based on value and effort.
Sometimes, the highest value opportunities aren’t the best ones to pursue first if the effort is too high — you want to aim for something that can be delivered in the next six to 12 months. Plus, Parinaz notes, your team might not be mature enough to take on more complex challenges yet.
“We didn’t pick the hardest problem, we picked an important problem that we knew we could do,” said Edgardo. “We wanted to take something that would show progress, but also was reasonably attainable.”
Companies should also keep in mind competitive advantages. “What are the trends in your market? What are the table stakes right now? How can you really think about the premium differentiation in your market?” said Parinaz. “What is so manual right now that you can really gain lots of efficiencies and improve your margins?”
For example, customer service can be tedious and take up a lot of time, meaning there are many opportunities for automation to make the service better and more efficient, said Edgardo.
“One of the things that we have focused on with ML is how do we make that process more efficient, so that the people focus on things that, frankly, humans are good at so that the machines can do what machines are good at.”
Overcoming data challenges
Data availability and quality can affect your AI products.
In our piece on conducting a data audit and ensuring you have clean data, Parinaz noted that the companies can run into challenges with data for a few reasons: the quality of data is low, or you just don’t have enough data yet that could adequately train an ML model.
To combat some of these data quality challenges, it’s important to make sure the data collection process is clean. Inconsistencies can be made worse by not having an automated way to collect data, making it “noisy,” said Parinaz. This leads to models that don’t perform well.
Once your company has collected the right amount of quality datasets, you should also spend some time ensuring that data is readily available for use among other teams, and for future AI products.
“We have terabytes of data, but is the data in a way that we can actually use? We have [invested time] in making sure that the data is available,” said Edgardo.
To ensure that your AI work has longevity, have metrics that actually measure the success of using AI. For example, you can use the number of minutes saved using AI as a metric, he noted.
“I always want to make sure that we have identified the business goal, and then we have a way of quantifying [success],” said Edgardo.
Overall, AI isn’t something you use without a clearly defined business problem and measure of success.
How to hire the right talent
After you have a better idea of your needs, your next challenge will be attracting the right talent to match the scale of your project. As a startup, it can be difficult to compete with larger companies’ compensation packages. To compete against larger, more established players in the employer marketplace, emphasize the impact, visibility, and upward mobility possible at your organization.
Georgian’s frameworks for building high-performing machine learning teams lay out best practices for each maturity level. As a startup at the beginning of your AI journey, your priority should be setting a vision with your leadership team, identifying and prioritizing opportunities for building AI into your product and conducting a data audit. These steps will set you up for success to then identify the skills and experience needed to create a hiring plan.
If you have some ML expertise in your teams, you can train the existing talent you have who are interested in learning more about AI. Parinaz notes that full-time product managers, for example, can spend part of their time training with engineers. Those product managers could eventually become part of your full-time AI team.
This event series is part of Georgian’s CoLab, our collaborative pre-investment program for early-stage startups. If you’re interested in attending future CoLab Connect events or learning more about CoLab, contact Conor Ross.
Read more like this
Why Georgian Invested in Island (Again)
Island, the developer of the Enterprise Browser, emerged from stealth in early…
Why Georgian Invested in Render
We are pleased to announce Georgian’s investment in Render’s $80M Series C…