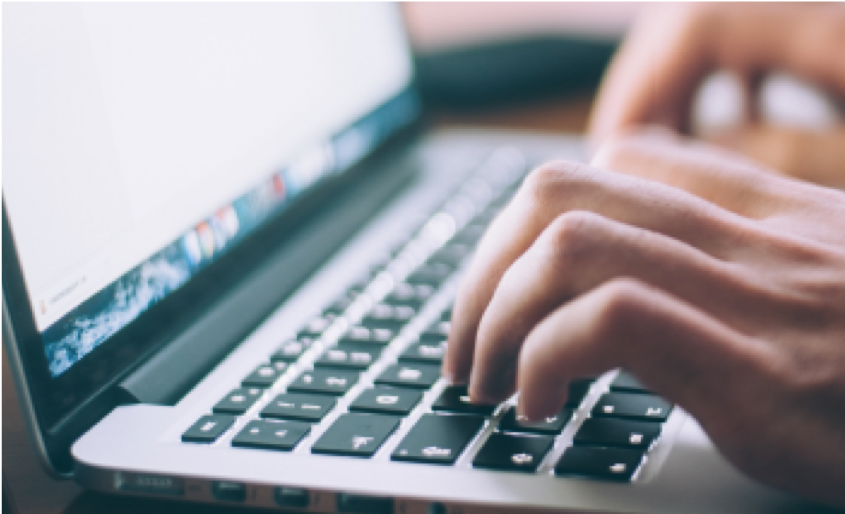
Case Study: How Turnitin Built a New Product with Trust at its Core
Turnitin created a new product that can analyze student essays to assess “contract cheating” while maintaining trust with its users by addressing fairness, reliability and explainability.
Turnitin works with universities and K-12 institutions to detect plagiarism in essays submitted to their service. Market research and customer interviews showed a new type of cheating was emerging, particularly in higher education, where students aren’t plagiarizing but they’re hiring somebody to write an essay for them, a trend called “contract cheating”.
To combat the problem, Shayne Miel, Principal Machine Learning Scientist, and the team at Turnitin devised a new product using Natural Language Processing and image analysis that can help academic investigators to detect whether a student’s submitted essay has been written by someone other than the student who submitted it. It highlights papers that are suspicious and merit closer inspection. Predictions are based on differences in writing style between a student’s previously submitted essays and the essay in question.
The team knew their customers would find this product valuable, but due to the sensitive nature of the problem, they had major concerns in three areas – fairness, reliability, and explainability. They knew that to build trust with users, they would have to address all three.
Root Out Bias
Turnitin reached out to the Georgian Impact team to explore how they could assist with this project. After several strategy meetings, they decided to work together on the fairness aspect.
First and foremost, Miel and the team wanted to be sure that any model or machine learning algorithm would treat all the students fairly since the impact of the product could be life-changing. It was important to create a test for bias to ensure there was a similar error rate for all groups, for example, gender, ethnicity, subject studied, native language.
To build fairness into the product, however, the team faced a challenge, because Turnitin does not collect any demographic information about students, so it was difficult to measure bias for any of these groups.
To find a solution, the Georgian R&D team looked at external sources of data to identify proxies that could be used for different demographics. This included interviews with subject-matter experts, knowledge from the Turnitin product team and a bias identification tool – FairTest. This tool enables users to identify and quantify associations between outcomes (predictions of the model or model error) and sensitive user attributes (e.g. gender, language proficiency).
“It was an interesting problem because sensitive attributes are domain-specific,” said Parinaz Sobhani, Director of Machine Learning at Georgian. “Whereas in many cases gender, ethnicity, or religion might be sensitive, in the case of Turnitin, native language became the most important issue.”
This is because a large number of contract cheating cases have been observed among English language learners, who struggle with the new language and so outsource the writing of their essays. Miel wanted to ensure that the high incidence of these types of cases did not bias the model to the disadvantage of non-native speakers.
Working closely with the Turnitin team, Sobhani and the Georgian R&D team developed a test framework to detect bias against any one subgroup. This allows the Turnitin team to monitor for bias and create a plan to address it.
Make Trust Measurable
In addition to ensuring fair outcomes for students, the Turnitin team also wanted to ensure that its users – academic investigators – continue to have high levels of trust in the predictions that the product makes. Trust is particularly important because identifying instances of potential contract cheating is not an exact science, and the team recognizes that to build trust, they have to set appropriate performance metrics and clearly communicate performance expectations to their users.
Turnitin, working with the Georgian R&D team, achieved this in two ways. First, they set the right metrics and performance thresholds to build confidence in the product’s reliability. Secondly, by focusing on explainability they ensured that users could understand why the prediction was made.
The team has also designed the product as a tool to make investigations more efficient, rather than as a means of automating student accusations. Machine learning can help show the investigators where to look, but it takes expert humans, ethics committees, and interviews with the student to actually determine what really occurred. This is where explainability is important.
Explain Yourself
Rather than labeling a student’s work as fraudulent or not, Turnitin’s product provides an estimate of how suspicious a student’s body of work is. To help the users of the products – academic investigators – trust the predictions and build their investigations, Turnitin’s solution highlights two types of evidence.
The first focuses on statistical features of the writing that can identify outliers in a student’s body of work. These guide the investigator towards the most anomalous papers. The second helps the investigator to build a case by highlighting specific areas in the essays that look suspicious. These features give the investigator a list of questions that they can use in interviews with the student. Both types of evidence were developed with the use of the product in mind, equipping the investigators with the information needed to complete their work.
The Georgian R&D team was a great partner and treated the problems we were working on with respect and enthusiasm. They provided thought leadership as we were defining the machine learning needs of the product and the fairness tool that will help us ensure our models are safe for use in production.
Shayne Miel, Turnitin
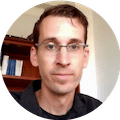
“For example,” Miel explained “if the student’s Flesch-Kincaid readability score is outside the range of most of their papers, that gets highlighted as suspicious. But you couldn’t ask a student a question about that, because they wouldn’t understand what a readability score means. There are other things that we’re looking at like spelling variants – British spelling versus American spelling – which do provide questions. If in one paper the student spells the word “centre” and in the other, it’s “center”, that provides a very specific question that investigators can ask.”
By addressing fairness, reliability and explainability and through the work with the Georgian R&D team, Turnitin has been able to build a valuable product that can earn the trust of its users while tackling a sensitive issue. The team is currently working on building the FairTest solution into their process, to ensure that every model is checked for bias before being released into the product.
Read more like this
Cloud Spend Management: A Guide for Startups
Over the past several months, CoLab executives and customers have told us…
How to Use OKRs to Unlock Your Company’s Potential
You’re probably familiar with OKRs — Objectives and Key Results. OKRs are…
Team Profile: Azin Asgarian, Applied Research Scientist
What do you work on at Georgian? As part of the R&D…